Keynote Talk by
Neil D. LAWRENCE
, University of Sheffield and Amazon
<
machine learning, computational biology, dimensionality reduction, Gaussian processes, probabilistic modelling
>
Title: Cloaking Functions: Differential Privacy with Gaussian Processes
Abstract : Processing of personally sensitive information should respect an individual’s privacy. One promising framework is Differential Privacy (DP). In this talk I’ll present work led by Michael Smith at the University of Sheffield on the use of cloaking functions to make Gaussian process (GP) predictions differentially private. Gaussian process models are flexible models with particular advantages in handling missing and noisy data. Our hope is that advances in DP for GPs will make it easier to ‘learn without looking’, i.e. gain the advantages of prediction from patient data without impinging on their privacy.
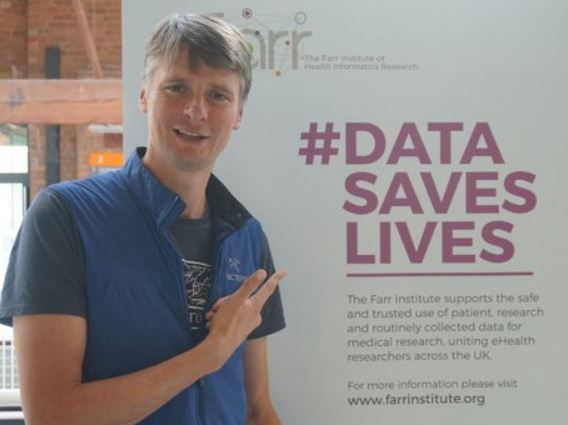
Image credit to Neil D Lawrence
Bio : Neil Lawrence is a Professor of Machine Learning and Computational Biology at the University of Sheffield. He holds a PhD in Computer science from Cambridge University and had a postdoctoral stay with Microsoft Research Cambridge. He has served as the Chair of the NIPS Conference, the premier Machine Learning conference in the world, and was the founding editor of the Journal of Machine Learning (JMLR) Research Workshop and Conference Proceedings. He is a fellow of the Royal Society in the working group for machine learning.
Neil is considered as one of the foremost experts on probabilistic modeling of real-world phenomena, specifically using Gaussian Processes. With his group, he is leading efforts to apply machine learning techniques for health informatics, particularly computational biology and personalized health.
More information: http://inverseprobability.com