IFIP – the I nternational F ederation for I nformation P rocessing is the leading multi-national, non-governmental, apolitical organization in Information & Communications Technologies and Computer Sciences, is recognized by the United Nations (UN) and was established in the year 1960 under the auspices of the UNESCO as an outcome of the first World Computer Congress held in Paris in 1959.
IFIP is incorporated in Austria by decree of the Austrian Foreign Ministry (20th September 1996, GZ 1055.170/120-I.2/96) granting IFIP the legal status of a non-governmental international organisation under the Austrian Law on the Granting of Privileges to Non-Governmental International Organisations (Federal Law Gazette 1992/174).
IFIP brings together more than 3500 scientists without boundaries form both academia and industry, organized in more than 100 Working Groups (WG) and 13 Technical Committees (TC).
The International Cross Domain Conference for Machine Learning & Knowledge Extraction CD-MAKE
is a joint effort of IFIP
TC 5 (Information Technology Applictions)
,
TC 12 (Artificial Intelligence)
, IFIP
WG 8.4 (E-Business: Multi-disciplinary research and practice)
, IFIP WG
8.9 (Enterprise Information Systems)
and IFIP WG
12.9 (Computational Intelligence)
and is held in conjunction with the International Conference on
Availability, Reliability and Security (ARES)
.
CD
stands for
C
ross-
D
omain and means the integration and appraisal of different fields and application domains (e.g. Health, Industry 4.0, etc.) to provide an atmosphere to foster different perspectives and opinions. The conference is dedicated to offer an international platform for novel ideas and a fresh look on the methodologies to put crazy ideas into Business for the benefit of the human. Serendipity is a desired effect, and shall cross-fertilize methodologies and transfer of algorithmic developments.
MAKE stands for MA chine Learning & K nowledge E xtraction.
Machine learning deals with understanding intelligence for the design and development of algorithms that can learn from data and improve over time. The original definition was “the artificial generation of knowledge from experience”. The challenge is to discover relevant structural patterns and/or temporal patterns (“knowledge”) in such data, which are often hidden and not accessible to a human. Today, machine learning is the fastest growing technical field, having many application domains, e.g. health, Industry 4.0, recommender systems, speech recognition, autonomous driving, etc. The challenge is in decision making under uncertainty, and probabilistic inference enormously influenced artificial intelligence and statistical learning. The inverse probability allows to infer unknowns, learn from data and make predictions to support decision making. Whether in social networks, recommender systems, health or Industry 4.0 applications, the increasingly complex data sets require efficient, useful and useable solutions for knowledge discovery and knowledge extraction.
A synergistic combination of methodologies and approaches of two domains offer ideal conditions towards unraveling these challenges and to foster new, efficient and user-friendly machine learning algorithms and knowledge extraction tools: Human-Computer Interaction (HCI) and Knowledge Discovery/Data Mining (KDD) , aiming at augmenting human intelligence with computational intelligence and vice versa.
Consequently, successful Machine Learning & Knowledge extraction needs a concerted international effort without boundaries, supporting collaborative and integrative cross-disciplinary research between experts from 7 (the magical number seven +/- 0) fields (image below – and 3-minutes MAKE-Video ), and see the inaugural paper of the new Machine Learning & Knowledge Extraction (MAKE) journal:
Holzinger, A., 2017. Introduction to MAchine Learning & Knowledge Extraction (MAKE). Machine Learning and Knowledge Extraction , 1(1), p.1. Available at: http://dx.doi.org/10.3390/make1010001 .
❶ DATA – Data science (data fusion, preprocessing, mapping, knowledge representation),
❷ LEARNING – Machine learning algorithms,
❸ VISUALIZATION – and visual analytics
❹ PRIVACY – data protection, safety, security, ethics, acceptance and social issues of ML
❺ NETWORK – graphical models, graph-based ML
❻ TOPOLOGY – geometrical machine learning, topological data analysis
❼ ENTROPY – time and machine learning, entropy-based ML
The goal of the CD-MAKE conference is to act as a Catalyst to bring together researchers from these seven areas in an cross-disciplinary manner, to stimulate fresh ideas and to encourage multi-disciplinary problem solving.
Last updated by A.Holzinger 13.01.2018 08:00 CET
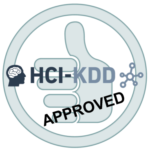