MAKE-Explainable AI (MAKE – eXAI)
CD-MAKE 2019 Workshop on explainable Artificial Intelligence
The preliminary workshop page under construction can be found here:
https://hci-kdd.org/make-explainable-artificial-intelligence-2019
GOAL
This catalysator-workshop aims to bring together international cross-domain experts interested in machine learning/AI to stimulate research, engineering and evaluation in and for explainable AI – towards making machine learning results transparent, re-enactive, comprehensible, interpretable, thus explainable, re-traceable and reproducible on demand, towards causality research.
Accepted papers will be presented at the workshop and published in the IFIP CD-MAKE Volume of Springer Lecture Notes (LNCS) and/or (if the application domain is health) as a journal contribution to the special 2019 collection “Explainable AI for medical informatics and decision making” in Springer/Nature BMC Medical Informatics and Decision support (MIDM) – see:
https://hci-kdd.org/special-issue-explainable-ai-medical-informatics-decision-making
There is also a possibility to submit extended versions of the conference papers to our MAKE journal:
https://www.mdpi.com/journal/make
All submissions will be peer reviewed by at least three experts – see authors instructions here: https://cd-make.net/authors-area/submission
BACKGROUND
Explainable AI is NOT a new field. Actually the problem of explainability is as old as AI and maybe the result of AI itself. While early expert systems consisted of handcrafted knowledge, which enabled reasoning over at least a narrowly well-defined domain, such systems had no learning capabilities and were poor in handling of uncertainties when (trying to) solving real-world problems. The big success of current AI solutions and ML algorithms is due to the practical applicability of statistical learning approaches in arbitrarily high dimensional spaces. Despite their huge successes their effectiveness is still limited by their inability to ”explain” their decisions in an human understandable and retraceable way. Even if we understand the underlying mathematical theories, it is complicated and often impossible to get insight into the internal working of the models, algorithms and tools and to explain how and why a result was achieved. Future AI needs contextual adaptation, i.e. systems that help to construct explanatory models for solving real-world problems. Here it would be beneficial not to exclude human expertise , but to augment human intelligence with artificial intelligence .
TOPICS:
In line with the general theme of the CD-MAKE conference of augmenting human intelligence with artificial intelligence , and Science is to test crazy ideas – Engineering is to bring these ideas into Business – we foster cross-disciplinary and interdisciplinary work including but not limited to:
- Novel methods, algorithms, tools, procedures for supporting explainability in AI/ML
- Proof-of-concepts and demonstrators of how to integrate explainable AI into workflows and industrial processes
- Frameworks, architectures, algorithms and tools to support post-hoc and ante-hoc explainability
- Work on causality machine learning
- Theoretical approaches of explainability (“What makes a good explanation?”)
- Philsophical approaches of explainability (“When is it enough, do we have a degree of saturation?”)
- Towards argumentation theories of explanation and issues of cognition
- Comparison Human intelligence vs. Artificial Intelligence (HCI — KDD)
- Interactive machine learning with human(s)-in-the-loop (crowd intelligence)
- Explanatory User Interfaces and Human-Computer Interaction (HCI) for explainable AI
- Novel Intelligent User Interfaces and affective computing approaches
- Fairness, accountability and trust
- Ethical aspects and law, legal issues and social responsibility
- Business aspects of explainable AI
- Self-explanatory agents and decision support systems
- Explanation agents and recommender systems
- Combination of statistical learning approaches with large knowledge repositories (ontologies)
Workshop Organizers:
Randy GOEBEL
, University of Alberta, Edmonton, CA (workshop co-chair)
Yoichi HAYASHI
,
Meiji University, Kawasaki, JP (workshop co-chair)
Freddy LECUE
, Accenture Artificial Intelligence Technology Labs, Dublin, IE and INRIA Sophia Antipolis, FR
Peter KIESEBERG
, Secure Business Austria, SBA-Research Vienna, AT
Andreas HOLZINGER
, Medical University Graz, AT (workshop co-chair)
Program Committee:
Jose Maria ALONSO
,
CiTiUS – University of Santiago de Compostela, ES
(1, 2)
Explainable AI, Soft Computing, Computational Intelligence, Fuzzy Logic, NLG, Data Science
Tarek R. BESOLD
Telefonica Innovation Alpha, Barcelona, Spain
(1,2)
Data Science, Artificial Intelligence, Computational Creativity, Knowledge, Explainable AI
Guido BOLOGNA
Computer Vision and Multimedia Lab, Université de Genève, Geneva, CH
Artificial Intelligence, Machine Learning, Computer Vision, Bioinformatics
Federico CABITZA
Università degli Studi di Milano-Bicocca, DISCO, Milano, IT
Human-Computer Interaction, Health Informatics, Decision Support, Information Quality, socio-technical systems
Ajay CHANDER
Computer Science Department, Stanford University and Fujitsu Labs of America, US
David EVANS
Computer Science Department, University of Virginia, US
Computer Security, Applied Cryptography, Multi-Party Computation, Adversarial Machine Learning
Pim HASELAGER
Donders Institute for Brain, Cognition, and Behaviour
, Radboud University, Nijmegen, NL
(2)
Artificial Intelligence, Cognitive Science, Explainable AI
Freddy LECUE
Accenture Technology Labs, Dublin, IE and INRIA Sophia Antipolis, FR
(1, 2)
Artificial Intelligence, Service Computing, Semantic Web, Knowledge Represenation, Explicative Reasoning
Daniele MAGAZZENI
Trusted Autonomous Systems Hub, King’s College London, UK
Artificial Intelligence, Explainable AI, Robotics, Planning, Autonomous Systems
Tim MILLER
School of Computing and Information Systems, The University of Melbourne, AU
artificial intelligence, human-agent interaction, explainable AI, AI planning
Huamin QU
Human-Computer Interaction Group & HKUST VIS, Hong-Kong University of Science & Technology, CN
data visualization, visual analytics, urban computing, E-learning, Explainable AI
Stephen K. REED
Center for Research in Mathematics and Science Education, San Diego State University, US
Cognitive Science, Cognitive Psychology, Problem Solving, Informatics
Marco Tulio RIBEIRO
Microsoft Research, Redmond, WA, US
Machine Learning
Marco SCUTARI
Instituto Dalle Molle di Studi sull’Intelligenza Artificiale, Lugano, CH
Bayesian Networks, Machine Learning, Software Engineering, Applied Data Analysis
Andrea VEDALDI
Visual Geometry Group, University of Oxford, UK
Computer Vision,Image Understanding,Machine Learning
Jianlong Zhou
Faculty of Engineering and Information Technology,University of Technology Sydney, AU
Transparent Machine Learning, Behaviour Analytics, Cognitive and Emotional Computing, Eye-tracking and GSR, Human Computer Inter
Christian BAUCKHAGE
Fraunhofer Institute Intelligent Analysis, IAIS, Sankt Augustin, and University of Bonn, DE
Pattern Recognition, Machine Learning, Web Science, Computer Games
Vaishak BELLE
Belle Lab, Centre for Intelligent Systems and their Applications, School of Informatics, University of Edinburgh, UK
Artificial Intelligence
Frenay BENOIT
Universite de Namur, BE
Machine Learning
Enrico BERTINI
CSE Department, NYU School of Englineering, New York University, US
(2, 3)
Visual Analytics, Information Visualization, User Interfaces
Aldo FAISAL
Brain & Behaviour Lab and Machine Learning Group, Imperial College London, UK
(1, 2)
Neurotechnology, Neuroscience, Machine Learning, Motor Control, Brain Science
Bryce GOODMAN
Oxford Internet Institute and San Francisco Bay Area, CA, US
Hani HAGRAS
Computational Intelligence Centre, School of Computer Science & Electronic Engineering, University of Essex, UK
Computational Intelligence, Fuzzy Logic, Ambient Intelligence, Artificial Intelligence, Intelligent Control
Barbara HAMMER
Machine Learning Group
, Center of Excellence & Faculty of Technology, Bielefeld University, DE
(1, 2)
Machine Learning, Data Mining, Neural networks, Bioinformatics, Theoretical Computer Science
Shujun LI
Kent Interdisciplinary Research Centre in Cyber Security (KirCCS), University of Kent, Canterbury, UK
Cyber Security, Human-Centric Computing, Digital Forensics, Multimedia Computing, Applications of AI
Brian Y. LIM
Department of Computer Science, National University of Singapore, SG
(1, 2)
Applied Machine Learning, Explainable AI, Pervasive Computing, Internet of Things, HCI
Luca LONGO
School of Computer Science, Technological University Dublin, IE
ESR (1, 2, 3)
Knowledge representation, artificial intelligence, decision making, HCI
Brian RUTTENBERG
Charles River Analytics, Cambridge, MA, US
Google scholar
Wojciech SAMEK
Machine Learning Group, Fraunhofer Heinrich Hertz Institute, Berlin, DE
Machine Learning, Interpretability, Deep Learning, Explainable AI, Robust Signal Processing
Gerhard SCHURZ
Düsseldorf Center for Logic and Philosophy of Science, University Düsseldorf, DE
Philosophiy of Science, Logic, Epistemology, Cognitive Science
Sameer SINGH
University of California UCI, Irvine, CA, US
Machine Learning, NLP and Information Extraction, Interpretability
Alison SMITH
University of Maryland, MD, US
Human Computer Interaction, Computational Linguistics, Machine Learning, Interactive Machine Learning, Human-in-the-Loop
Mohan SRIDHARAN
University of Auckland, NZ
Human-robot collaboration, Knowledge representation and reasoning, Machine learning, Computational vision, Cognitive systems
Janusz WOJTUSIAK
Machine Learning and Inference Laboratory, Center for Discovery Science and Health Informatics, George Mason University, Fairfax, US
(1, 2)
Artificial Intelligence, Machine Learning, Health Informatics
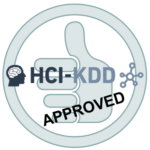